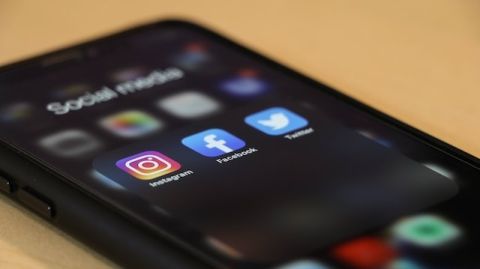
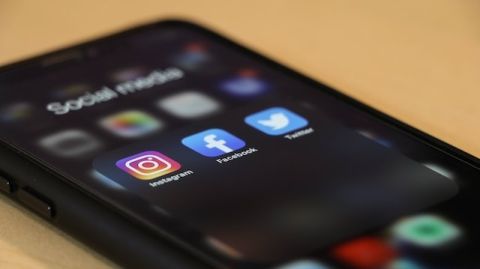
Experts warn current machine learning models are missing microaggressions and subtle cognitive warfare online
2 February 2023
7
A NATO Strategic Communications Centre of Excellence (StratCom COE) report has warned many of the artificial intelligence (AI) tools used to monitor social media posts are too literal and struggle to detect subtle hostile messaging and misinformation.
Many machine learning models allow platforms, companies and governments to estimate the emotion of posts and videos online.
However, a week before the World Artificial Intelligence Cannes Festival (9-11 February) a team of experts say the majority of these AI-based systems rely on understanding the sentiment behind a message, which isn’t as clear cut as first thought.
Their study published as part of a collaboration between the University of Portsmouth and a NATO Strategic Communications Centre of Excellence research report, explores trends in AI. It outlines the limitations of these open-source sentiment strategies and recommended ways to improve them.
The goal of microaggressive text online is to attack an individual, group, organisation or country, in a way that is difficult to spot when analysed by AI.
Subtle microaggressions are dangerous on social media platforms as they can often resonate with people of similar beliefs and help spread toxic or hostile messaging.
Dr Alexander Gegov, Reader in Computational Intelligence and Leader of the University of Portsmouth
Dr Alexander Gegov, Reader in Computational Intelligence and Leader of the University of Portsmouth team working the research for NATO StratCom COE, said: “Subtle microaggressions are dangerous on social media platforms as they can often resonate with people of similar beliefs and help spread toxic or hostile messaging.”
“Estimating emotions online is challenging, but in this report, we demonstrated that there are many ways we can enhance our conventional pre-processing pipelines. It is time to go beyond simple polar emotions, and teach AI to assess the context of a conversation.”
The authors say the Google Jigsaw’s emotion classifier is an interesting addition to analysing polar emotions online, but its classifiers are unable to distinguish between readers’ responses to toxic comments or someone spreading hate speech.
They found a different approach, known as the rhetorical structure theory (RST), is a more robust and effective way of analysing microaggressions. In a way, it mimics how brains unconsciously weigh different parts of sentences by assigning importance to certain words or phrases.
For example: ‘Today is pretty bad’ and ‘That’s a pretty dress’ both contain the positive word ‘pretty’. But ‘pretty’ may also intensify the sentiment of the words around it, e.g. ‘bad’.
Clearly analysing text alone is not enough when trying to classify more subtle forms of hate speech.
Djamila Ouelhadj, Professor in Operational Research and Analytics at the University of Portsmouth
“Clearly analysing text alone is not enough when trying to classify more subtle forms of hate speech”, explained the research’s co-leader Djamila Ouelhadj, Professor in Operational Research and Analytics at the University of Portsmouth.
“Our research with the NATO StratCom COE has proposed some recommendations on how to improve the artificial intelligence tools to address these limitations.
“Learning how an individual has put together a message offers a rich, untapped information source that can provide an analyst with the ‘story’ of how and why the message was assembled.
“When analysing messages and tweets from offensive or anti-West groups and individuals, for example, the RST model can tell us how radicalised a group is, based on their confidence on the topic they’re broadcasting.
“It can also help detect if someone is being groomed or radicalised by measuring the level of insecurity the person displays when conveying their ‘opinion’.”
The team has produced an array of data sets to understand microaggressions, and tested them using English and Russian text.
They pulled a sample of 500 messages in the Russian language from a Kremlin-linked Telegram channel discussing the Ukrainian war, and analysed their hostility levels using the Google Jigsaw model.
The translated text scored lower in toxicity compared to the original Russian documents. This highlighted that when messaging is translated from its original language by AI, some of the toxic inferences are missed or overlooked. The effect might be even stronger when analysing microaggression, where the hostility isn’t as obvious.
To overcome this, the paper says online translators can be fine-tuned and adapted to countries and region-specific languages.
Subtle hostile social media messaging is being missed by artificial intelligence tools
Dr Gundars Bergmanis-Korāts, Senior Expert at NATO StratCom COE, said: “The governments, organisations, and institutions of NATO and allied countries must address current AI challenges and focus on adjustments to local language specifics in order to ensure equal IEA capabilities.
“Military and government organisations leverage machine learning tools to detect, measure, and mitigate disinformation online, and measure the effectiveness and reach of communications. Therefore, understanding audiences by analysing the context of communication is crucial.”
Last year, the US set aside more than half a million dollars to be spent in developing an artificial intelligence model that can automatically detect and suppress microaggressions on social media.
Dr Gegov added: “Often the overall essence of social media messaging is hidden between less relevant sentences, which is why manual filtering and post-processing steps on platforms are necessary.
“This will probably not change overnight, but while there’s no one ‘tool that does it all’, we explore some simple tricks that data analysts and AI enthusiasts can do to potentially increase the performance of their text-processing pipelines.
“We also encourage social media monitors to become more transparent about what systems they’re currently using."